The 1,2,3 of Predictive Analytics
With the advent of cookie-less browsers and strict privacy regulations, predictive analytics can aid marketers in finding better results. Here's how
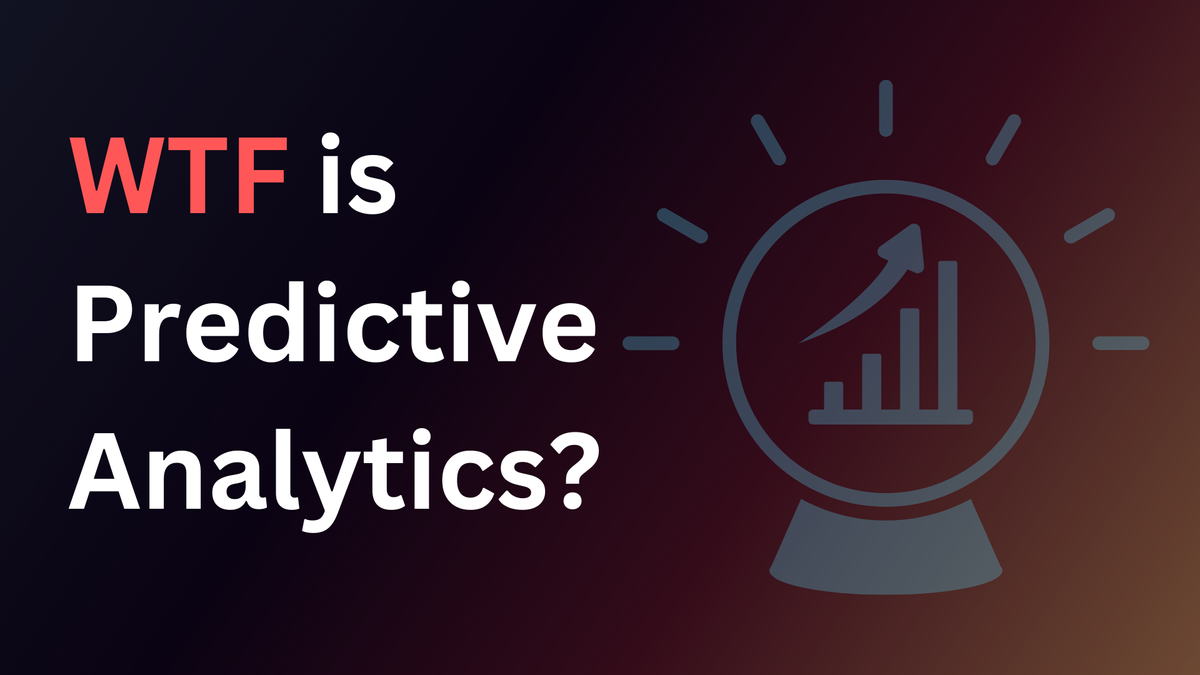
If you're a marketer, one of your biggest concerns (especially this year) is most probably about the phasing out of third-party cookies from Chrome. This is just one development—though admittedly a really big one—in an online world that has seen a slew of privacy first measures over the last three-four years.
Such developments coupled with privacy regulations and growing consumer awareness have made—and will continue to make—traditional targeting methods way more difficult to execute. This shift necessitates a new approach to understanding and reaching customers effectively without compromising their privacy.
1. Privacy Makes Targeting Tougher
Privacy regulations like GDPR in Europe and CCPA in California underscore a growing demand for privacy-first practices, compelling marketers to find alternative ways to personalize ads without infringing on user privacy. The traditional reliance on vast pools of personal data for targeting is no longer viable in the long run; it's time to look at more innovative solutions that can deliver effective ad experiences, while respecting user privacy.
2. Predictive Models Can Help
Enter predictive analytics, an approach that uses AI and machine learning to forecast future consumer behavior based on historical data. Unlike traditional targeting methods that rely heavily on personal data, predictive models can identify patterns and trends in anonymized data sets, offering insights into consumer preferences and behaviors without the need for personal identifiers. These models analyze past interactions, such as purchase history, content engagement, and search behavior, to predict how similar users and audiences will likely respond to different advertising strategies in the future.
Predictive analytics can enable marketers to segment their audience more effectively — especially based on interactions — personalize messaging, and optimize campaign timings and channels, all while adhering to privacy standards.
In a cookie-less world, here's how predictive analytics can be employed using first-party data:
- Personalization: Tailoring website content, product recommendations, and marketing messages based on individual customer interactions and preferences identified through first-party data.
- Customer Segmentation: Using behavioral patterns and transaction data to segment customers into different groups for targeted marketing campaigns.
- Churn Prediction: Identifying customers who are likely to churn based on their engagement levels and transaction history, allowing for proactive retention strategies.
- Lifetime Value Prediction: Estimating the future value of customers to prioritize marketing efforts and resource allocation.
- Optimized Customer Journey: Analyzing customer journeys and interactions to streamline and improve the buying process; this in turn will enhance customer experience and increase conversions.
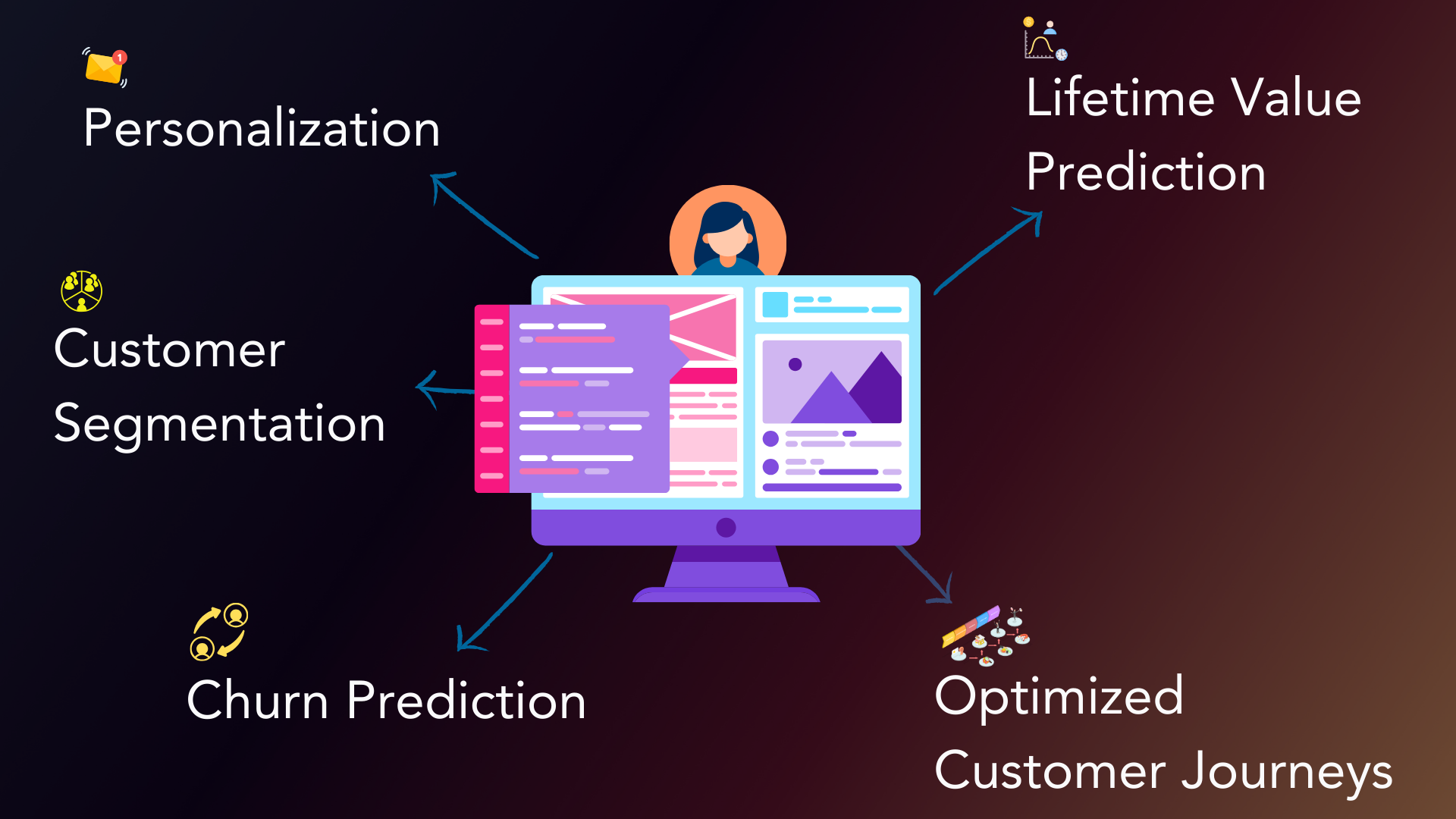
So even if you're interested in using a predictive model, how do you actually think about implementing this?
3. Implementing Predictive Analytics
If you've just started to think about putting a predictive model together, there are a few key, and challenging, steps that need to be carefully thought out while either building or using a predictive analytics tool:
- Data Collection and Preparation: Begin by gathering anonymized data from your digital channels. This data should be cleaned and organized to ensure it's ready for analysis.
- Model Development: If you're building your own model, then be prepared to set aside some budget and experience to utilize machine learning algorithms to develop predictive models. This process involves selecting appropriate algorithms, training them on your historical data, and fine-tuning them to improve prediction accuracy.
- Integration and Application: Integrate the predictive models with your advertising platforms. Use the insights gained from these models to inform your ad targeting strategies, content personalization, and campaign optimizations.
- Continuous Learning and Optimization: As with any AI system, predictive models improve with more data. Continuously monitor your campaign performance, feeding new data back into the models to refine your predictions and strategies over time.
These steps are just a starting point, and actually building and implementing a predictive system has many finer details to analyze. And importantly, the transition towards predictive analytics requires a mindset shift from reactive to proactive marketing.
Some Resources
In the new era of digital privacy, AI-driven predictive analytics offers a powerful solution for marketers seeking to personalize their digital ads without infringing on user privacy. As technology continues to evolve, so too will the ways in which we can ethically and effectively reach our audiences. And predictive analytics can be a valuable tool for this.
Here are some resources that might help you get some clarity:
1. 6 top predictive analytics tools for 2024, George Lawton, TechTarget
2. Predictive Analytics: Predicting Customer Behavior to Improve ROI, Angela Hausman, Market Maven
3. Predictive Analytics for Sales and Marketing, Dana Khoury Eid, UniAthena